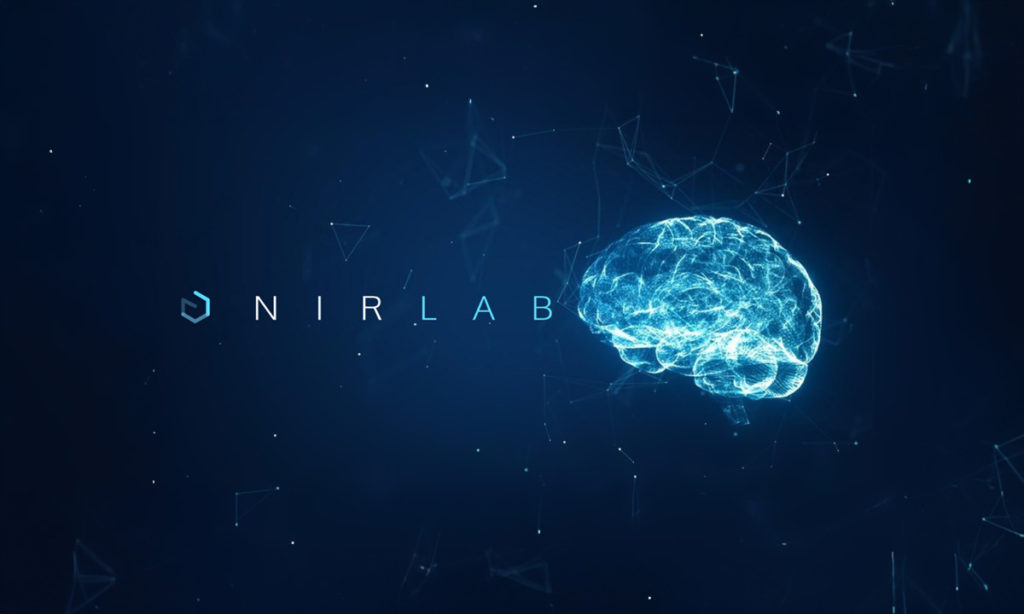
Introduction
In today’s fast-paced industrial landscape, the demand for efficient and accurate material analysis is greater than ever. Industries such as pharmaceuticals, agriculture, and public safety require reliable, real-time data to ensure quality, safety, and performance. Traditional lab testing is being replaced by Near-Infrared (NIR) Spectroscopy, a technology enhanced by machine learning (ML) that enables faster and more insightful material analysis. NIRLAB is at the forefront of this innovation, implementing these advanced technologies across diverse fields to deliver immediate, actionable insights.
Material science serves as the foundation for quality and innovation, providing essential knowledge about the molecular composition of materials. This understanding is crucial for enhancing safety, effectiveness, and overall product integrity.
NIR Spectroscopy and the Role of Machine Learning
Near-Infrared Spectroscopy (NIR) stands out in the field of material analysis for its ability to provide rapid, non-destructive insights. By measuring how materials absorb near-infrared light, NIR can reveal complex molecular details without extensive sample preparation. However, NIR spectra often feature broad, overlapping absorption bands caused by complex molecular vibrations, creating data that is rich but noisy.
Unlike other spectroscopic methods like Infrared or Raman, which yield distinct, narrow bands, NIR’s overlapping spectra present a unique challenge for data interpretation. NIRLAB addresses this complexity by leveraging machine learning to streamline and enhance data analysis, turning NIR spectroscopy into a practical tool for real-time material insights.
Machine learning is essential for interpreting the intricate data patterns generated by NIR spectroscopy. ML algorithms handle large datasets with overlapping spectral data, uncovering patterns and correlations that would be impossible to detect manually. Hence, this combination of NIR and ML enables real-time, accurate material analysis, which is vital for industries that rely on fast decision-making and precision.
Key Machine Learning Techniques for Enhanced NIR Spectroscopy
To maximize the effectiveness of NIR spectroscopy, several key machine learning techniques are employed, each designed to address the unique challenges posed by complex spectral data:
- Pattern Recognition in Overlapping Bands: Machine learning models trained on extensive datasets can “deconvolute” overlapping bands, revealing the specific spectral signatures of different components within a sample. This technique is especially valuable in complex samples, like pharmaceuticals and animal feed, where NIRLAB’s models can differentiate between various overlapping substances.
- Feature Extraction and Dimensionality Reduction: NIR data often contains vast amounts of information, much of it redundant. ML techniques like Principal Component Analysis (PCA) and Partial Least Squares (PLS) reduce data to its most informative features, speeding up and refining analysis. In feed analysis, for instance, NIRLAB can isolate critical nutritional data, such as protein or fat content, ensuring a targeted approach.
- Noise Reduction and Data Filtering: Real-world NIR measurements are often affected by environmental noise and sample variability. Machine learning algorithms filter out this noise, enhancing signal clarity and improving data reliability. For example, NIRLAB’s technology ensures high precision in narcotics detection, where accurate material identification is essential.
- Quantitative Analysis: Machine learning enables NIR to go beyond basic identification, offering precise quantitative measurements for industries where exact composition is crucial. In pharmaceuticals, NIRLAB’s ML-driven NIR can measure chemical purity, composition, and concentration, providing the accuracy required for regulatory compliance.
NIRLAB’s Applications of NIR Spectroscopy Across Industries
NIRLAB harnesses the power of NIR spectroscopy and machine learning across multiple industries to address diverse needs and enhance product quality and safety.
- Pharmaceuticals: NIRLAB employs advanced NIR and machine learning technologies to analyze counterfeit medicines and accurately determine the active pharmaceutical ingredients (APIs) present in them. This capability is essential for ensuring drug safety, effectiveness, and compliance with regulatory standards.
- Agriculture: In the agricultural sector, NIRLAB provides real-time analysis of crop quality, such as assessing sugar content in sugarcane or determining protein and fat levels in fish. These analyses help farmers optimize harvests and ensure high-quality produce for the market.
- Narcotics Detection: Accurate identification of illicit substances is vital for public safety. NIRLAB’s advanced NIR technology enables precise substance detection, assisting law enforcement in effectively managing and controlling narcotics.
With the integration of machine learning, NIRLAB is harnessing the potential of NIR spectroscopy to address the unique needs of various industries, delivering faster and more reliable results for both businesses and public sectors. Through these innovative applications, NIRLAB is paving the way for portable, real-time material analysis, enabling professionals to make informed, data-driven decisions across diverse settings.
As machine learning and artificial intelligence continue to evolve, NIRLAB is actively exploring new applications in areas such as quality control in food processing, environmental monitoring, and the assessment of raw material quality. These advancements promise to unlock even more possibilities for enhancing material analysis in the future.
Conclusion
The integration of NIR spectroscopy and machine learning is transforming material analysis, addressing the growing demand for accuracy, speed, and scalability in modern industries. Portable, AI-powered NIR devices are replacing heavy, costly lab infrastructure, enabling instant, real-time analysis and decision-making. As NIRLAB continues to innovate and expand these capabilities, industries can expect increased precision, broader applications, and faster, more accessible insights.
With advancements in AI and NIR technology, NIRLAB is leading the way toward a future where material science is more efficient, actionable, and integrated into the point of need. Whether in pharmaceuticals, agriculture, public safety, or environmental management, the combination of NIR devices and machine learning promises to enhance quality, safety, and sustainability across industries worldwide.
To explore more about spectroscopy and discover the full capabilities of NIRLAB’s technology, we invite you to read our other informative articles, here. Additionally, for personalized inquiries, please don’t hesitate to reach out to us at contact@nirlab.com.